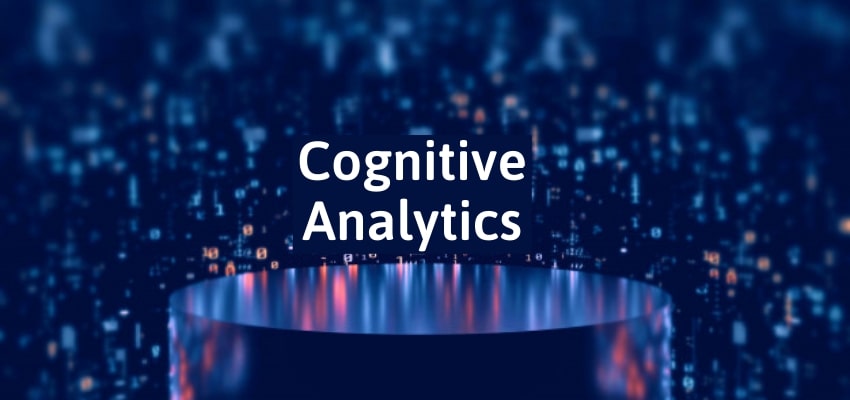
Cognitive Analytics blends artificial intelligence, machine learning, and human-like reasoning to handle data in a more insightful way. This approach transforms unstructured information into meaningful patterns that go beyond standard statistical methods.
Many organizations have embraced Cognitive Analytics to stay ahead of the curve, make smarter decisions, and detect trends hidden beneath massive datasets.
Whether dealing with business forecasts, language understanding, or image recognition, Cognitive Analytics stands out as a key tool in the modern data-driven world. It not only refines how data is interpreted but also reshapes how patterns are understood and acted upon.
Understanding Cognitive Analytics
Cognitive Analytics involves algorithms and techniques that try to mimic human thought processes. Traditional analytics relies on descriptive or predictive models built around raw data.
Cognitive methods introduce reasoning layers that evaluate context, semantics, and intent. A system of this kind uses knowledge graphs, machine learning frameworks, and language comprehension to generate well-rounded insights.
A standard analytics pipeline analyzes data for patterns and correlations. It might find seasonality or identify groups in the data. Cognitive Analytics, however, goes beyond that by interpreting context and deriving meaning from language.
A regular model might spot a spike in customer inquiries. A cognitive approach can dig into why those inquiries happened and what specific topics customers raised.
Another appealing aspect lies in its continuous learning. These models do not stop once they reach a conclusion. They refine their output when given updated information, adjusting future predictions. This adaptive quality helps organizations respond quickly to changing conditions.
For example, a bank that processes loan applications may keep adjusting how it judges creditworthiness as new behavior patterns appear. This dynamic nature sets Cognitive Analytics apart from systems that remain static.
Deep language comprehension stands at the center of many cognitive tools. Machine translation, chatbots, and sentiment analysis all benefit from interpreting words in context.
Past analytical methods treated text as a bag of words. Cognitive Analytics tries to detect intent and emotion behind those words. It builds a richer model of user sentiment and needs. That can lead to better customer service approaches and more accurate product recommendations.
In short, Cognitive Analytics refines how data is processed. It sharpens raw information into logical connections, reveals hidden insights, and offers a more intuitive decision-making foundation.
How Cognitive Analytics Works
Cognitive Analytics draws upon multiple disciplines to achieve its goals. Machine learning is a major part of the equation. It includes neural networks and other models capable of spotting patterns in large datasets.
Yet there is more at play than brute-force computation. Natural Language Processing (NLP) is another essential ingredient. It allows machines to interpret text or speech with greater insight.
Data Ingestion
- Pulls data from social media, documents, sensors, or user surveys
- Converts messy data into standardized formats
- Cleans and prepares information for advanced analysis
Machine Reasoning
- Identifies logical connections between different data sources
- Uses knowledge graphs or semantic networks to reflect real-world linkages
- Incorporates context, which goes beyond raw numbers
Learning Mechanisms
- Employs supervised or unsupervised techniques to detect patterns
- Adjusts future results by learning from ongoing data feedback
- Ensures that models stay relevant over time
Output and Decision Support
- Provides predictions or recommendations that reflect evolving circumstances
- Delivers insights through dashboards, automated alerts, or text summaries
- Bridges the gap between analytics output and business actions
Organizations that deploy Cognitive Analytics often set up workflows linking various stages together. One example is an e-commerce firm capturing customer interactions from website logs and social media.
That data flows into cleaning routines before advanced machine learning algorithms generate user segmentation.
By applying language comprehension, the system recognizes the tone behind reviews or complaints. Executives receive these insights in an accessible format, driving more targeted marketing or product enhancements.
This entire cycle underscores the key purpose of Cognitive Analytics: to produce reasoning, not just results. The data pipeline goes beyond raw metrics. It interprets, understands, and learns, leading to outputs that resemble human decision-making processes.
Key Components of Cognitive Analytics
A variety of technologies merge under the Cognitive Analytics umbrella. Each part has a specialized function, yet they complement one another to deliver deeper insights. Below are the primary building blocks that enable these solutions:
1. Machine Learning & Deep Learning
Modern machine learning provides classification, clustering, and recommendation methods. Deep learning extends these by applying layered neural networks designed for tasks like image recognition or language translation. Together, they uncover subtle relationships in data, which could be overlooked by simpler approaches.
2. Natural Language Processing (NLP)
NLP focuses on interpreting human language. This feature allows systems to analyze sentiments in reviews or detect intentions in support tickets.
By examining word usage, syntax, and tone, NLP paves the way for a more complete interpretation of text-based data. Chatbots, automated summarizers, and sentiment analyzers depend heavily on NLP engines.
3. Knowledge Graphs
Knowledge graphs represent relationships between entities in a structured way. They store information as nodes and edges, mapping out how data elements link to each other.
A system using knowledge graphs could, for instance, connect a product name to its manufacturer, user feedback, and even relevant regulatory standards. This mesh of connections assists in making more informed inferences.
4. Cognitive Computing
This facet of Cognitive Analytics is about simulating reasoning approaches similar to the human mind. It covers aspects such as logic-based problem-solving and contextual understanding.
A cognitive computing layer can incorporate domain-specific knowledge to refine recommendations. For instance, a healthcare application that reviews patient data might consider lab results along with established medical guidelines to suggest a suitable diagnosis path.
5. Continuous Learning and Adaptation
Cognitive models do not remain static. They take in new data and update their internal parameters to keep pace with shifting trends. This agility is crucial in fast-changing areas like financial markets or e-commerce. It ensures that the model’s suggestions stay accurate, even as user behavior or external conditions evolve.
These components blend together to form a synchronized engine for data-driven insights. Each module performs a unique function, yet the combination leads to outcomes that mirror how humans reason and learn, only at machine speed and scale.
Applications of Cognitive Analytics
Cognitive Analytics offers a broad range of uses across different sectors. It goes beyond traditional reporting by offering context-driven insights and continuous refinement. Below are some prime examples where Cognitive Analytics makes a difference:
1. Customer Service & Engagement
- Chatbots and Virtual Assistants: These tools use NLP to interpret queries and provide quick answers. They can even pick up on emotional cues or detect subtle hints of user frustration.
- Sentiment Analysis: By scanning social media posts or survey feedback, organizations can sense how consumers feel. This leads to more precise marketing and product development strategies.
- User Profiling: Cognitive methods go past simple demographic splits and capture behavioral patterns. Businesses target their audience more accurately and predict churn risks.
2. Healthcare
- Medical Diagnosis: Systems can study a patient’s records, lab results, and research papers to offer insights on possible treatments.
- Patient Monitoring: Wearable devices collect real-time vitals that feed into cognitive systems. They catch irregular patterns early, flagging potential concerns for doctors.
- Drug Discovery: Large volumes of research data benefit from machine reasoning. Patterns emerge that might accelerate the search for new therapies.
3. Finance & Banking
- Fraud Detection: Cognitive analytics uncovers anomalies by interpreting transaction data in context. It spots unusual patterns that standard methods might overlook.
- Customer Risk Assessment: Models become more nuanced by including non-traditional data, such as social media or alternative credit histories. This approach can refine how loans or insurance policies are issued.
- Trading Algorithms: High-frequency trading systems thrive on advanced analytics. A cognitive layer can integrate market news, investor sentiment, and real-time updates for better forecasting.
4. Manufacturing & Supply Chain
- Predictive Maintenance: Cognitive models process sensor data to forecast equipment failure. Downtime shrinks and productivity improves.
- Quality Control: Computer vision and deep learning analyze production line images. They spot flaws and ensure consistency.
- Supply Chain Optimization: Data on weather, logistics, and consumer demand helps in adjusting inventory levels. This approach prevents overstock or shortage issues.
5. Cybersecurity
- Threat Intelligence: Cognitive engines scan network logs, user behavior, and known malware patterns to predict possible breaches.
- Real-Time Alerting: As soon as suspicious activity appears, the system flags it. In some cases, it can initiate protective steps automatically.
- Security Policy Development: Analytics-driven risk assessments help security teams shape guidelines that adapt to changing threats.
These applications demonstrate how Cognitive Analytics reshapes decision-making. It uncovers patterns that can guide more thoughtful actions and strategies in a world overflowing with data.
Challenges and Considerations
Though Cognitive Analytics brings many benefits, several issues need attention before jumping in. Some revolve around data, while others concern ethical implications or resource constraints.
1. Data Quality and Volume
Large datasets feed cognitive models, and the quality of that data matters. Systems might produce unreliable insights if the content is biased or incomplete.
Filtering, cleaning, and verifying data become serious tasks. High-performance hardware may also be necessary because processing such huge volumes can be computationally heavy.
2. Ethical and Regulatory Factors
Many industries face strict rules on how data is stored and used. Financial and healthcare sectors must pay close attention to privacy. Overstepping boundaries with consumer data can lead to significant penalties. Data anonymization and encryption can help, but consistent oversight remains essential.
3. Talent and Resources
Cognitive Analytics involves complex mathematics, advanced programming, and specialized knowledge of machine learning frameworks. Hiring or training personnel who can work at this level is often challenging.
Budget constraints or limited executive backing may block progress. Meanwhile, open-source tools are evolving, offering smaller organizations a path to begin without massive costs.
4. System Complexity
Cognitive models have many moving parts, including neural networks, knowledge graphs, and more. Maintaining and scaling these systems demands thorough planning. In addition, interpretability can be tricky.
Black-box algorithms might make predictions that are hard to explain. Stakeholders who prefer transparent decision-making processes may resist methods they cannot fully understand.
5. Security Vulnerabilities
Cognitive systems are not immune to attacks. Malicious actors might feed them false data or exploit loopholes to corrupt outputs. Rigorous testing, patching, and encryption help reduce this risk. Ongoing system audits and zero-trust approaches also protect sensitive information.
Strategies for Successful Implementation
Transitioning to Cognitive Analytics requires a structured plan. Blindly deploying new tools rarely leads to success. The following suggestions aim to smooth this process:
- Clear Objectives: Start with a concrete goal. Examples include improving product recommendations or reducing fraud losses. Quantify success metrics ahead of time, such as expected cost savings or improved accuracy rates.
- Choose the Right Tools: Evaluate frameworks that can handle machine learning, NLP, or knowledge graphs. Consider whether open-source libraries meet the needs or if a proprietary solution fits better.
- Data Governance: Implement rules that maintain quality across all data sources. Keep track of data lineage. Show how data moves through the organization and undergoes transformations.
- Scalable Infrastructure: Set up systems that can expand without losing performance. Hybrid or cloud-based architectures often handle large data demands well.
- Security and Compliance: Encrypt sensitive information and ensure user privacy policies comply with legal requirements. Establish monitoring solutions that spot unusual system or user behaviors.
- Ongoing Training and Maintenance: Provide technical teams with refresher courses on evolving machine learning techniques. Keep models up to date by regularly testing them and feeding fresh data.
- Cultural Acceptance: Encourage employees to adopt a data-first mindset. Communicate the purpose of these solutions so staff members trust and embrace them.
Every organization differs in structure and goals. A multi-phase rollout might work best. Deploying a small pilot project can produce early insights, which then guide wider adoption.
Future Prospects of Cognitive Analytics
Cognitive Analytics continues to change rapidly. Quantum computing, for instance, could push processing speeds to unseen levels, making sophisticated models run faster. Another potential growth area lies in explainable artificial intelligence (XAI). This approach provides more transparent reasoning behind predictions, building greater trust among decision-makers.
Edge computing also merges well with Cognitive Analytics, especially in settings that rely on real-time data processing. Drones, autonomous vehicles, or advanced IoT devices need immediate feedback with minimal latency. Running cognitive models closer to the data source can serve that purpose.
Adoption might also expand through user-friendly platforms. Low-code or no-code solutions lower the barrier to entry, allowing domain experts to experiment without deep coding skills. As the technology becomes more accessible, smaller businesses and nonprofits may also seize these opportunities to tackle unique challenges.
Overall, advancements in hardware, software, and methodology promise an exciting path forward. The rate of change underscores the ongoing value of staying informed and adaptable in this space.
Frequently Asked Questions (FAQs)
Q1. How does Cognitive Analytics differ from standard analytics?
Traditional analytics focuses on descriptive or predictive tasks using historical data. Cognitive approaches introduce context and reasoning, interpreting data in ways that approximate human thought patterns.
Q2. Why is data quality crucial for Cognitive Analytics?
Systems learn and adapt based on the content fed to them. Unreliable or inaccurate data can produce skewed outputs. High-quality information yields dependable insights.
Q3. Can small businesses benefit from Cognitive Analytics?
Yes. Scalability and open-source tools allow smaller operations to pilot projects. Starting with focused objectives helps manage costs and deliver measurable results.
Q4. Which skills are needed for implementing Cognitive Analytics?
Knowledge of machine learning, data engineering, and domain expertise is key. Familiarity with programming languages like Python and frameworks that support deep learning is also common.
Q5. Does Cognitive Analytics replace human decision-making?
It aims to enhance, not replace. Automated models highlight trends and suggest actions. Human judgment remains relevant for ethical questions or complex strategic choices.
Conclusion
Cognitive Analytics marks a new approach to understanding data. Through machine reasoning, language comprehension, and continuous refinement, it offers insights that go beyond raw figures.
Organizations use it to optimize decision-making, find hidden opportunities, and adapt quickly in dynamic environments. From automated customer support to complex medical diagnostics, this forward-thinking methodology serves a broad range of needs.
As technology advances, Cognitive Analytics will likely keep evolving, offering solutions that model decision-making in a more natural and adaptive manner.
Also Read: