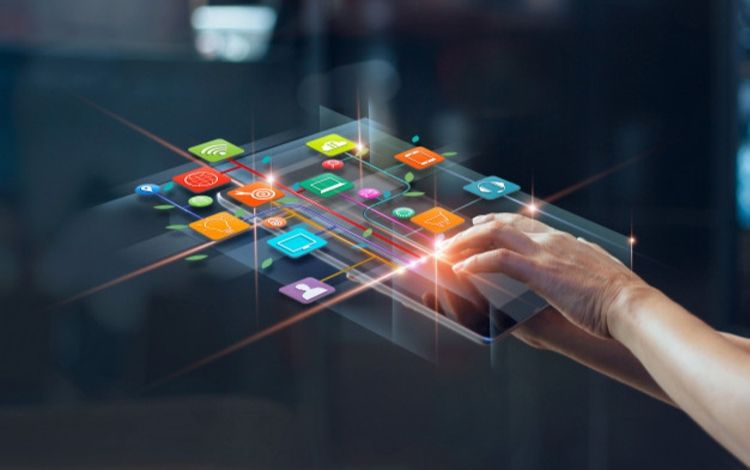
Bigdata has become a massive force shaping retail decision-making worldwide. Large volumes of transactions, social media updates, and demographic details feed countless databases.
Global e-commerce sales surpassed $6 trillion in 2024, which underscores the vast scope of digital shopping. Large retailers process billions of transactions each year, feeding sophisticated models that outline shopper preferences.
Leaders in the industry draw insights from these enormous datasets to streamline product recommendations, pricing, and operational management. Customers expect personalized experiences, and data-driven solutions offer sharper ways to meet evolving demands.
Brands like Amazon are well-known for robust recommendation engines that drive a significant portion of sales. Grocery chains monitor weekly point-of-sale data to predict produce demand and reduce waste.
Some apparel companies rely on Bigdata to pick color palettes that resonate with target groups. Each real-world success story shows how data insights guide better decisions and stronger consumer engagement.
Sophisticated analytics are transforming both small and large retailers by providing meaningful knowledge about spending trends. An exploration of Bigdata reveals how it touches every link in the retail chain.
Understanding Bigdata in Retail
Bigdata refers to digital information whose volume, velocity, and variety grow beyond what traditional tools can handle. Retailers handle many data streams, including point-of-sale records, online browsing behavior, loyalty program details, and supply chain metrics.
Each piece of information offers clues about how shoppers think and act. The complexity often arises when multiple sources merge, especially in an era when customers move across channels at lightning speed.
Organizations have embraced advanced analytics systems to extract trends from this ocean of data. Modern servers and distributed computing methods parse large files while statistical models highlight purchasing patterns.
Machine learning techniques have become central, examining consumer tastes and anticipating future decisions. A thorough understanding of data creation and analysis leads to higher profitability and smoother processes.
Skipping data analysis can lead to missed sales opportunities, poor inventory forecasts, and unplanned expenses. Harnessing Bigdata establishes a strong competitive edge in today’s retail scene.
Types of Bigdata in Retail
Data flows into retail environments from multiple entry points. Each source offers value when captured and processed accurately:
- Transactional Data: Details from every sale, refund, and exchange reflect shopper activity. Time stamps, payment methods, and items purchased create a historical record of trends.
- Customer Behavior Data: Website clicks, mobile app usage, and in-store beacons track how individuals shop, linger, and respond to promotions.
- Social Media and Online Reviews: Opinions shared on platforms reveal feedback about a brand and its offerings. Positive and negative comments can spread quickly, influencing public perception.
- Loyalty Program Data: Membership initiatives generate records about frequency of visits, basket size, and engagement. Personalized deals rely on these patterns.
- Supply Chain and Inventory Data: Movement of goods from warehouses to stores holds clues about demand and operational costs. Delays or overstocks become easier to manage when real-time metrics are in place.
Each category intersects with others, creating a web of insights. Clean, accurate data leads to actionable findings. Old or inconsistent information skews analytics and drains resources. Efficient storage, flexible retrieval, and ongoing updates keep information fresh and relevant.
Role of Bigdata in Customer Experience
Clients want seamless, consistent interactions whether browsing online or stepping into a physical store. Bigdata strengthens that experience by offering tailored recommendations and personalized coupons.
Data platforms detect browsing habits, brand preferences, and spending thresholds. E-commerce sites often present custom product suggestions after spotting patterns in purchase history. Repeat shoppers might also receive updates when favorite items go on sale.
Such personalization extends to offline settings too. Loyalty cards and mobile apps capture in-store behavior. Insights from those channels lead to better floor layouts or improved product placement.
Predictive analytics also determine when certain promotions resonate. In many cases, marketing messages shift based on weather forecasts or holiday seasons, and Bigdata tools track the impact of each campaign.
Shoppers who feel understood tend to remain loyal and share positive feedback with friends and colleagues.
Managers appreciate the clarity Bigdata offers in real time. Quick dashboards reveal sudden surges in demand, prompting timely action. Product restocking, staff scheduling, or pricing tweaks can happen swiftly when analytics spot upward or downward changes.
That rapid response addresses consumer expectations around fast service and consistent inventory availability. Data science, in that sense, underpins a better retail atmosphere.
Predictive Analytics in Retail
Predictive analytics stands as a method of forecasting future shopper behavior and industry events. Algorithms observe patterns, correlations, and outliers within large datasets. Historical sales records might indicate that certain items sell better on weekends.
External data, such as economic indicators or local events, can also influence outcomes. The ability to predict these behaviors helps merchants plan stock levels, coordinate marketing, and set promotions well in advance.
Clustering methods group shoppers by traits like age, location, or brand loyalty. Collaborative filtering explores how one person’s tastes align with others, leading to better product suggestions. Classification models determine the probability of cart abandonment or churn.
Regression techniques detect the factors that best explain store foot traffic. Each approach relies on thorough data preparation and continuous monitoring of results. Swift adjustments become possible when a model indicates shifts in consumer habits.
Bigdata Tools and Techniques in Retail
Technological solutions handle both data storage and advanced analytics. Cloud platforms store massive volumes of structured and unstructured data securely.
Distributed frameworks process complex computations rapidly, avoiding the bottlenecks of older architectures. Many retailers invest in software that can run predictive models in near-real time.
Common Tools and Approaches
- Hadoop and Spark: Used to handle large datasets, partition tasks, and run algorithms at scale.
- Machine Learning Libraries: TensorFlow, PyTorch, and scikit-learn aid in classification, recommendation systems, and forecasting.
- NoSQL Databases: Offer flexibility for storing images, text, and logs without the constraints of traditional table structures.
- APIs and Data Integration: Connect different business systems and facilitate seamless data sharing among departments.
Retailers often combine these components to handle batch processing for historical analysis and streaming analytics for real-time decisions.
Multinational chains might blend internal server farms with public cloud services. Smaller outlets can benefit from user-friendly analytics dashboards that require minimal technical knowledge.
Future Trends in Retail Bigdata
Artificial intelligence holds promise in detecting customer sentiment and real-time reactions. Voice commerce stands poised to expand further as technologies like smart speakers mature.
Micro-influencer campaigns gain momentum when Bigdata identifies niche communities. Augmented reality might enrich virtual fitting rooms, with analytics tracking user engagement to refine these services.
Seamless cross-channel shopping will remain a central focus. Buyers jump from mobile apps to physical stores, and integrated systems can keep all data synchronized.
The power of Bigdata will only grow as new devices produce more streams of information. Trends suggest continued investment in machine learning initiatives that automate various workflows, from distribution to post-purchase support.
Conclusion
Bigdata continues to reshape how stores promote, price, and deliver products. Layers of digital information shed light on what drives consumer loyalty. Sales forecasts gain greater precision, and personalized marketing campaigns resonate more effectively.
Decision-makers can move with agility, guided by metrics that reflect genuine trends. Transformations happen swiftly when data-driven insights enter discussions around shelf space, customer experience, and future growth.
Retailers who prioritize careful analysis and continuous innovation stand ready to succeed in a data-saturated market.