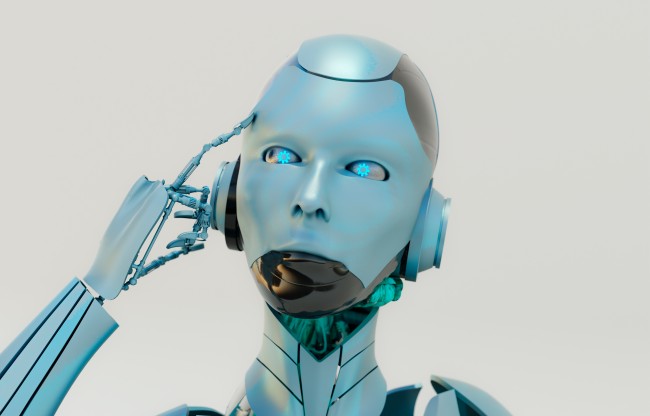
Simply put, a machine learning model’s health is the stage of effectiveness and performance of a machine learning model. It shows how accurately or precisely the model’s outputs match expectations. It shows the system’s reliability in performing tasks with the planned outcomes.
It is crucial to have continuous monitoring to ensure that an ML model is in optimum health. However, monitoring can be difficult without visibility. Some ML developers have difficulty keeping track of vital data, stats, and metrics. Consider the tips below to achieve better ML model health visibility.
Set clear performance metrics
It is important to clearly understand what your ML project is all about so you can set the necessary metrics for performance evaluation. Different projects have different goals and areas of concern.
Therefore, you can’t just follow generic model performance evaluation templates. Instead, find out which metrics are useful for your project. The most common metrics are accuracy, precision, recall, F1 score, and AUC-ROC.
Take advantage of monitoring tools
There’s a lot to be thankful for about modern technology. In particular, there is a suitable tool for virtually every need you can think of. When it comes to machine learning model monitoring, you can easily find a good tool or solution.
Aporia, for example, offers an ML observability platform that makes it easy to keep track of ML model health and facilitates the implementation of improvements.
A quick Google search will lead to dozens of useful monitoring solutions compatible with different AI development platforms. Many tools can enable the real-time tracking of an ML model’s accuracy, precision, recall, and other metrics.
Use visualization techniques
Reviewing various metrics and evaluating them can be a tedious and exhausting process. Sometimes, you end up thinking that you have poor visibility over your machine learning model’s health even when you already have access to all of them.
This can happen because of data fatigue. It helps to have a refreshing presentation of information about the performance of your system, like having them visualized. Visualization can show data clearly and concisely, making it easier to see data patterns and trends.
Ensure continuous data logs
Project logs are more helpful than you likely think they are. They provide information that allows you to spot problems more easily. The information they bear is also important in coming up with solutions to problems in your system.
Logs related to input data, output predictions, and especially system errors can help you keep track of performance issues and identify the right solutions.
Audit your system regularly
ML model auditing is similar to model performance monitoring. However, in most cases, auditing entails more actions to ensure system integrity. It does not only keep track of performance metrics.
It is also designed to keep the project in line with various standards, including standards for security. Auditing encompasses examining input data, predictions, algorithms, biases, errors, and vulnerabilities.
Ascertain that you have full visibility over your ML model’s health. Doing so does not only help you complete a project as intended. It also helps you see issues and needs you may have overlooked.