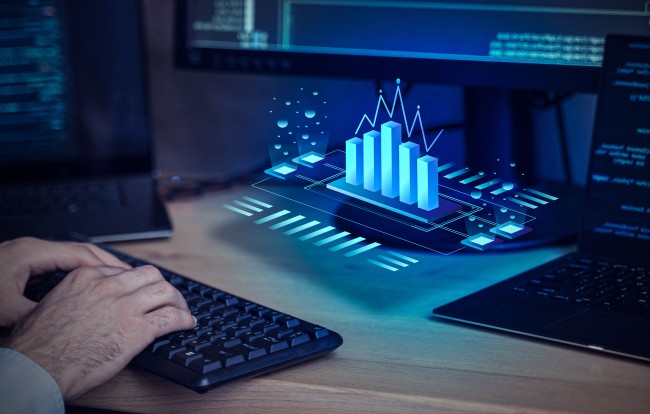
Modern enterprises run on data. From fueling operations to running sales campaigns, data lies at the heart of every task a company executes. As companies collect more data, their risk of exposure to adverse impacts increases.
Hackers have become highly sophisticated these days, and enterprises are vulnerable. Enterprises must prioritize data risk management, but few companies look beyond cybersecurity when thinking of data.
Here in this article we will discuss few reasons why it is important for companies to streamline data risk management. Let’s dive in.
1. Prevent data breaches
Cybersecurity is the most obvious reason for enterprises to safeguard their data. Thankfully modern companies have prioritized security as a product feature for a long time now. Despite prioritization, companies could do with a few improvements.
Static security postures are still common. A static posture refers to a company installing a platform and expecting it to handle every threat. However, modern cyber threats need a dynamic handling approach.
Companies must use continuous monitoring tools to constantly test their software and discover vulnerabilities. Once discovered, security teams can patch weaknesses before attackers discover them.
A combination of the right tools and automated testing like this will help companies create a dynamic security posture that prevents any risk of data loss.
2. Ease data migrations
Data migrations occur pretty frequently within enterprises. Whether upgrading legacy systems or building new layers on top of them, data moves around a lot, between a lot of systems, and this movement introduces gaps in data management.
One of the biggest risks with data migration is the involvement of sub-applications in the process.
For instance, when moving data from one location to another, data dependencies must move too. Subsystems might introduce additional logic to comply with the new location, complicating migration timelines.
The move itself tends to be complex due to the Waterfall-like approach to migration companies use. They usually wait for one system to finish processing its portion of the application chain before migrating that data downstream.
Automation and data management tools are a great solution to this problem. By automating jobs, companies can schedule migration dependencies efficiently, saving them time and money in the long run.
3. Draw the right insights
Here’s a thing that most companies miss about data: It gets stale if left unused or not updated. The problem is companies collect so much data these days, they don’t understand what’s in their databases.
Analytics built on top of such data will result in odd conclusions, leading to potential financial loss. Enterprises rely on analytics to power several business processes. Timely data reviews will help them stay on top of data quality, ensuring they generate the right insights.
The key to high data quality is timely data maintenance and reviews. Teams using analytics must review their data for validity and conduct a technical analysis. Is the team storing too many unnecessary files?
Are their data models reflective of how data is stored? Is there too much obsolete data in the system? Companies usually sweep such questions under the rug. Carrying outdated data for far too long.
While these outdated data increase costs, they also corrupt analytics. Companies also forget about such data and create duplicate information, which leads to even more confusion.
When decommissioning front-end systems, enterprises must plan to migrate or decommission back-end data too. This needs collaboration between the business and technical teams. As the volume of unstructured data grows, businesses must prioritize these processes to avoid data bloat.
4. Ensure correct data extraction
Companies have used several automated benefits like character recognition and robotic process automation to speed up internal processes. For instance, invoice processing platforms can read data from paper invoices, automatically apply cash, and create accounting journal entries.
While these automated processes save companies time, they create substantial data risk that needs managing. Where will companies store these unstructured data for translation? And how will they design processes to store that translated data?
Where will the raw data live and for how long should companies retain it? These questions don’t have easy answers, but companies should review their storage and retention policies to figure them out. Often, unstructured data leads to huge insights, so deleting them after translation might not make sense.
Another issue that complicates these processes is that companies usually don’t understand what they have till later. For instance, a company might be sitting in a goldmine of customer data but not understand it due to it being unstructured.
Retention policies reduce the risk of companies passing on these datasets and failing to draw insights.
Conclusion:
Companies have prioritized collecting data but spend comparatively less time minimizing the risks associated with it. The tips in this article will help companies get the most out of their data while minimizing risks.