Modern organizations collect enormous volumes of data about their clients and stakeholders, like markets, vendors, and many more. Businesses depend on the collected data from different directions to help them make critical daily decisions—yet the quality of their data analysis varies greatly.
This is due to the need for more resources or know-how to sift through millions of data points in various forms and sources to glean insights from the collected data for business value creation unless they have an in-house resource. Businesses use data discovery to help them transform this data into valuable insights.
Data discovery involves analyzing data using visual tools to help non-technical executives identify novel patterns and trends. Data discovery helps identify the pertinent data insights and convey them to the business users to enhance operations.
It further provides an analysis by product, category, and area, allowing for making data-driven decisions. Professionals from all departments will be able to make wise business decisions.
Let us walk you through what data discovery is and how it impacts businesses—but before we start, let us dig deeper into knowing the difference between data discovery and data exploration.
Understanding data discovery and data exploration
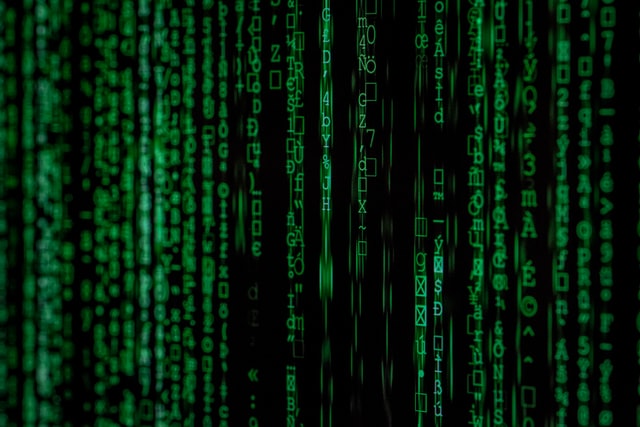
To comprehend the data better, data scientists apply cutting-edge analytics and statistics during the initial stage of data analysis, known as data exploration.
Following data preparation, they explore the data to determine which elements will contribute to illuminating the answers they seek. Data exploration must be viewed as a data filtration or narrowing procedure.
Data scientists will go through what they need to work on by using data exploration to see the different data sets. They use this to create a framework for the proper queries to ask while retrieving data.
Data exploration happens first in the sequence after data has been processed for additional analysis. Then you examine data sets to see which elements will aid your quest for the right solutions.
On the other hand, Once you are aware of the data that helps in your search for the solution, you sometimes go deep into the data to pinpoint the precise elements that hold the key to the solution and work out how to present the solution to the business teams.
Data discovery looks for patterns or trends in data. In this instance, data scientists are well aware of the topics they wish to address and the best places to hunt for the data necessary to do so. When those data sets have been located, you will have to go deeper to learn more details—that is data discovery.
What is data discovery?
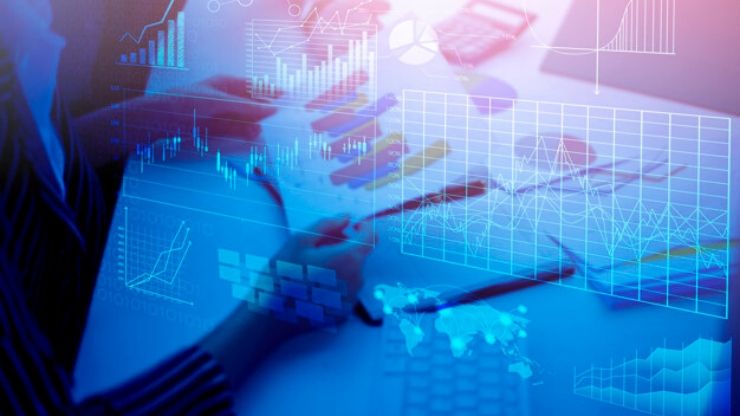
Data collected from many sources to find trends and patterns that help businesses identify and categorize critical and sensitive data is called data discovery.
The analyzed data is administered with more openness and for meaningful objectives. Businesses can execute sophisticated analytics and draw insightful conclusions from data using smart data discovery.
Data discovery helps by:
- Providing a means of making your data clear, accessible, and user-friendly
- Finding different insights on possibilities to provide value to your business
- Clean and prepare data, uncover underlying trends and connections, and provide insights without user input
- Creating comparable high-value business results to your corporate operations
- Reducing risk exposure from abuse by using data protection and abiding by privacy regulations
- Check for and assure higher data accuracy and increase cost efficiency significantly
A clear data research and discovery definition helps businesses to aggregate data from many sources, including external third-party data, and make better decisions for their company.
As a result, while conducting data discovery, you probably are not always sure what you’re searching for—instead, you just are looking for trends and outliers to help you understand your data better. Business users do not need to create complex models to leverage data discovery.
Most businesses who employ data discovery do it as a feature of their business intelligence (BI) software, which gives them a comprehensive perspective of their organizations in an easy-to-understand dashboard or visual style.
Data discovery, the initial phase of a data governance journey, gives a business the data knowledge it requires to create new goods and services, maximize data usage, and safeguard data from risk exposure.
The result makes it possible to collect more significant amounts of data from today’s modern organizations, opening up more options for new income streams.
How is data discovered?
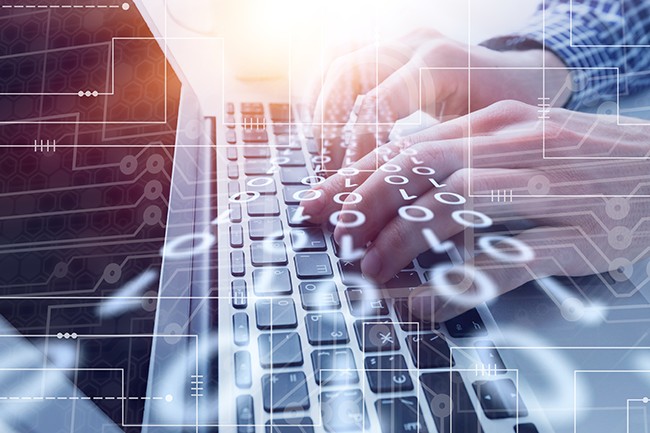
Data discovery is a continuous process that involves collecting and evaluating data from many sources and is frequently used to uncover trends, patterns, and errors in the data.
It is similar to organization-based analytical methods. It necessitates a series of actions that businesses utilize as a framework to comprehend their data.
Data discovery is an incremental method, so businesses will keep collecting, analyzing, and refining their strategy over time by learning from their findings and the input of business stakeholders.
The processes taken throughout the data discovery process constantly cooperate to offer hidden insights, possible security vulnerabilities, and visual mapping.
Data discovery involves the following steps:
Step 1: Determine needs and compile data
A defined goal, such as eliminating a problem, is the first step in effective data discovery. This entails thinking about the valuable information types while keeping open to the unexpected realization that will arise.
For instance, an insurance business will analyze its site data to lower bounce rates for fresh leads. Since the data stream will only provide part of the picture, it is crucial to aggregate and integrate data from many sources to succeed.
Step 2. Clean and prepare data
This is the labor-intensive data discovery component and a crucial component of its value. Data is reorganized during the data preparation step to make data discovery’s visualization, and analysis stages go more quickly.
Organizations often decrease the noise in their data and gain better results from their data analyses by cleaning the data and preparing it for analysis.
Without preparation, the data will be too cluttered to effectively reveal any hidden business insights. Data quality within the datasets under investigation is cleaned and merged during data preparation.
In addition to various tools for discovery and categorization, there are many different kinds of software available today that offer data preparation. These automated methods will eliminate outliers, harmonize data formats, find null values, and maintain a consistent level of data quality.
Step 3. Visualization of data
The data moves on to the visualization step once every data point has been converted into a standardized and understandable format. Data visualization is arguably the most crucial phase of data discovery since it forms the basis of AI-based business intelligence.
By presenting the prepared data in visual representations like charts, graphs, maps, etc., visual data discovery, also known as data mapping, gives business specialists access to deeper insights and practical platforms for visual analysis.
These visual aids show the main patterns discovered in the dataset being processed due to data mining, preparation, and sorting.
Step 4. Analyze the data
Business executives get a full picture of their operations and resolve the operational problems that prevent efficiency by combining data from several departments, integrating it with external data, and cleaning it for analysis.
The data is evaluated to be condensed and arranged into an easily understandable manner when the mapping and visualization process is finished.
Summary in the form of descriptions is frequently used during the analytical process. Instead of a spreadsheet, the analysis seeks to explain the effects of visualization and offers descriptive statistics that make the data more understandable and nearly narrative in nature.
Data discovery is an iterative process—organizations must continually improve upon this process to fully realize their data’s potential.
Why is data discovery important?
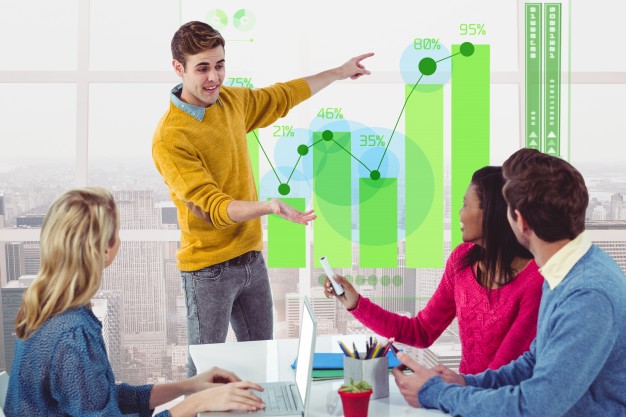
With data discovery organizations are better equipped with tools, knowledge and the ability to analyze their valuable data resources and unleash new insights.
Data discovery is becoming significantly popular as more and more businesses view their data as an asset. The data has the potential to make organizations stand out from the competition by using the data they gather about their clients and operations.
They will be in a position to use this insight to achieve a competitive edge through data discovery, whether it takes the shape of product innovation, improved consumer experiences, or efficiency improvements.
Data discovery helps process improvement for comprehending the data that the company owns, where it is stored, who will access it, where it will access it, and how it will be communicated.
Data discovery is important because it ensures:
- Sensitive data tracking, identification, and categorization
- Monitoring of data access and activity in real-time and in-depth
- Risk control and legal compliance
- Data scalability across teams
- The context-based categorization of data
- Transparent data that is clean and reused
Data discovery acts as a link between organizations’ goals and their customer’s demands in today’s data-rich business environment.
Data discovery helps turn raw data collection into data intelligence that drives value creation, ultimately enhancing strategies for a profitable business.
Data discovery will help you save time and resources while also providing departmental and employee-level insights that will help you identify the cause of compliance problems.